What Is Artificial Intelligence Technology and How Does It Work in Business in the USA?

Artificial Intelligence (AI) is no longer just a buzzword; it is a transformative force that is changing how businesses operate in the United States. From automating customer service to optimizing logistics, AI is improving efficiency, lowering costs, and creating new opportunities. But how does it function, and why has it become such an essential element of modern business strategy?
In simple terms, AI refers to the simulation of human intelligence in machines programmed to think and learn. These systems are capable of analyzing data, recognizing patterns, solving problems, and even making decisions with minimal human intervention. In the fast-paced business environment of the U.S., where time equates to money, AI helps organizations remain competitive and innovative.
Let’s examine the basics of AI technology and its applications across various industries in the U.S
.Introduction to Artificial Intelligence (AI)
Defining Artificial Intelligence
Artificial Intelligence refers to the development of computer systems that can perform tasks typically requiring human intelligence. These include things like understanding natural language, recognizing speech or images, making decisions, and translating languages.
AI is built on the foundation of data—lots of it. The more data a system has, the more effectively it can learn and improve. Think of it as giving the system experiences from which it can conclude, much like a human does. But instead of years of life experience, AI consumes millions of data points in seconds.
There are different levels and types of AI, ranging from basic automation to advanced systems that can simulate reasoning. Today’s businesses often use Narrow AI, which is designed for specific tasks like chatbots or recommendation engines. However, the ultimate goal is General AI, which would be able to perform any intellectual task that a human can do.
A Brief History of AI Development
AI development services has been since the 1950s when pioneers like Alan Turing and John McCarthy laid the groundwork. Early systems were rule-based and limited, but with the rise of computing power and data availability, AI took off in the 2010s.
In the U.S., companies like IBM, Google, and Microsoft have been at the forefront of AI research, with breakthroughs in deep learning and natural language processing accelerating adoption across industries.
AI’s growth was further fueled by cloud computing, which made high-powered AI processing accessible to businesses of all sizes—not just tech giants. Today, even small businesses can implement AI tools to streamline operations and improve customer experience.
Types of Artificial Intelligence
Narrow AI vs. General AI
The distinction between Narrow AI and General AI is essential for understanding how businesses utilize the technology.
- Narrow AI is task-specific. It excels at one job—like voice recognition or playing chess. It’s what powers Siri, Alexa, Google Assistant, and the majority of AI tools used in businesses today.
- General AI is still a concept more than a reality. It would be capable of performing any cognitive function that a human can. While it sounds futuristic, researchers are making strides toward it, though we are likely decades away from true general intelligence.
In business contexts, narrow AI is far more practical and useful. It’s mature, well-developed, and can deliver measurable value almost immediately.
Machine Learning, Deep Learning, and Natural Language Processing
AI encompasses several subfields:
- Machine Learning (ML): This is the most common type of AI in use. It allows systems to learn from data and improve without being explicitly programmed. Businesses use ML for predictive analytics, fraud detection, and customer segmentation.
- Deep Learning: A subset of ML that uses neural networks with many layers (hence “deep”). It’s especially good at recognizing images, video, and natural language. Think facial recognition or real-time language translation.
- Natural Language Processing (NLP): NLP enables machines to understand, interpret, and generate human language. It’s what powers chatbots, sentiment analysis tools, and language translation apps.
Together, these technologies make AI powerful and versatile, with applications across virtually every business sector in the U.S.
How AI Works: The Technical Side
Data Collection and Processing
AI systems thrive on data. The first step in any AI project is gathering the right kind of data—structured or unstructured, historical or real-time.
For instance, a retail business might collect transaction histories, customer feedback, and social media mentions. This data is cleaned and organized so the AI system can use it to learn and make predictions.
But collecting data isn’t enough. It must be relevant, accurate, and sufficiently large. Poor data leads to poor predictions—a concept known as “garbage in, garbage out.”
Algorithms and Training Models
Once the data is ready, it’s used to train algorithms. These algorithms are sets of rules that guide the AI in identifying patterns and making decisions.
Training involves feeding the algorithm massive datasets so it can learn what to look for. For example, a fraud detection model might be trained on thousands of past transactions to learn what fraudulent activity looks like.
The better the training, the more accurate the AI. It’s an iterative process: the model makes predictions, developers evaluate the accuracy, and the model is refined repeatedly.
AI Deployment and Real-Time Decision Making
After training, the AI model is deployed into a real-world environment. This could mean integrating a chatbot on a website or using AI to scan resumes in HR software.
Modern AI can even make real-time decisions. For example, credit card companies use AI to instantly approve or deny transactions based on fraud probability. Retailers use AI to dynamically adjust prices based on demand, competition, and inventory levels.
This is where AI shines in business—delivering insights and actions at a scale and speed no human team could match.
Key Applications of AI in Business
AI in Customer Service
Customer service is one of the biggest beneficiaries of AI. Chatbots, virtual assistants, and automated help desks provide 24/7 support, reduce wait times, and handle thousands of queries simultaneously.
These systems use NLP to understand customer intent and ML to improve their responses over time. They’re cost-effective and scalable, especially for businesses that operate across multiple time zones.
In the U.S., companies like Bank of America (Erica) and Capital One (Eno) have implemented AI-powered virtual assistants with great success, saving millions in operational costs.
AI in Marketing and Sales
AI has completely revolutionized how marketing and sales teams operate in the United States. It enables brands to target their audiences more precisely, personalize customer interactions, and predict buying behavior more accurately than ever before.
One of the most popular uses of AI in marketing is predictive analytics. These models analyze past customer data to predict future behaviors, helping marketers craft more effective campaigns. Instead of relying on guesswork, marketers now have insights on what product a customer might want, when they might want it, and what messaging will resonate most.
AI also powers personalization engines—the tech behind those “You might also like” or “Recommended for you” features on e-commerce platforms like Amazon and Netflix. These systems use real-time data to recommend products based on browsing history, location, purchase history, and even the behavior of similar users.
In sales, AI can score leads by analyzing factors like engagement history and demographic data to predict which prospects are most likely to convert. This helps sales teams focus their efforts on high-value leads, improving efficiency and increasing close rates.
Chatbots and virtual sales assistants can respond to inquiries, offer product recommendations, and navigate customers through the sales process—minimizing the reliance on human intervention and significantly enhancing the speed of the sales process.
AI in Human Resources and Recruitment
Hiring the right talent is critical to a company’s success—and AI is making this process smarter and faster. In HR departments across the U.S., AI is being used for resume screening, candidate matching, and interview scheduling.
One of the biggest benefits AI brings to recruitment is the ability to screen thousands of resumes in minutes, flagging the top candidates based on specific criteria like skills, experience, and education. Tools like HireVue and Pymetrics go a step further by using AI to assess candidates’ facial expressions, speech patterns, and responses in video interviews to evaluate soft skills and cultural fit.
AI is also helping companies reduce unconscious bias in hiring. By anonymizing resumes or relying on data-driven algorithms, HR teams can focus more on qualifications and less on potentially biased factors like name or background.
In employee engagement and retention, AI tools analyze feedback from surveys, performance reviews, and internal communications to identify trends that might lead to dissatisfaction or turnover. This allows managers to proactively address issues and create a more supportive work environment.
AI in Finance and Risk Management
In the finance sector, AI is not just a luxury—it’s a necessity. Financial institutions across the U.S. use AI for everything from fraud detection to portfolio management, credit scoring, and algorithmic trading.
AI models can spot suspicious transactions by comparing them against patterns of fraudulent activity. These systems flag anomalies in real time and alert security teams immediately—drastically reducing the risk of financial loss.
Credit scoring is another key area. Traditional credit checks are often rigid and limited, but AI can analyze a wider range of factors—like social media behavior, spending habits, and payment history—to provide a more nuanced assessment of creditworthiness.
In investing, AI-powered robo-advisors offer personalized investment advice and portfolio management at a fraction of the cost of human advisors. Tools like Betterment and Wealthfront are popular in the U.S., offering algorithm-driven financial planning that’s accessible to everyone.
AI also plays a critical role in risk assessment and compliance. By scanning massive datasets, AI can identify potential regulatory breaches or operational risks that humans might miss—helping firms stay compliant with financial laws and avoid penalties.
Industry-Wise Use of AI in the USA
AI in Healthcare
The healthcare industry is arguably one of the most impacted by AI in the U.S., with applications that range from diagnostics and treatment planning to patient monitoring and drug discovery.
AI algorithms can now interpret medical images like X-rays, MRIs, and CT scans with accuracy levels that rival or surpass human doctors. These tools help radiologists detect anomalies like tumors or fractures much faster and more accurately.
Virtual health assistants are providing preliminary diagnoses and health advice, reducing the burden on medical professionals. AI-powered chatbots can answer common patient questions, schedule appointments, and even remind patients to take their medications.
In drug development, AI is speeding up the process by predicting how different compounds will behave, identifying potential side effects, and suggesting modifications—all before a single clinical trial begins.
Hospitals are also using AI for operational efficiency. AI systems help manage inventory, predict patient admissions, and allocate staff more effectively, leading to cost savings and improved patient care.
AI in Retail
Retail businesses in the U.S. are using AI to personalize shopping experiences, manage inventory, and improve customer service. It’s especially valuable in both e-commerce and brick-and-mortar operations.
In online retail, AI analyzes customer behavior to recommend products, tailor marketing emails, and even adjust pricing dynamically based on demand, competition, and individual user behavior.
In physical stores, AI powers smart shelves that alert staff when products are running low or misplaced. Facial recognition and heat-mapping technologies help store managers understand customer behavior and optimize store layouts.
Customer support is also enhanced through AI chatbots that answer FAQs, handle returns, and guide customers through purchases—improving satisfaction and reducing costs.
Retail giants like Walmart, Target, and Amazon are heavily invested in AI to stay ahead of trends, streamline operations, and deliver more value to customers.
AI in Manufacturing
Manufacturers are using AI for predictive maintenance—sensors monitor equipment and signal when maintenance is needed, preventing costly downtime. AI also powers quality control systems that detect defects on the production line faster and more accurately than humans.
Robotics integrated with AI perform tasks like welding, painting, and assembly, increasing productivity while maintaining precision and safety.
AI in Transportation and Logistics
AI is driving the future of transport with autonomous vehicles, smart traffic management, and optimized delivery routes. Logistics companies like FedEx and UPS use AI to predict delivery times, reroute trucks in real-time, and minimize fuel consumption.
Warehouse automation—using robots and AI systems—is speeding up order fulfillment and reducing human error. Even airlines are leveraging AI for dynamic pricing and predictive maintenance.
How AI Works in the Business Environment
Data Collection and Processing
The journey of AI in a business setting begins with data—lots of it. AI systems thrive on data, whether it’s customer behavior, transaction histories, website clicks, or even weather forecasts. This raw data is collected from various sources like CRMs, social media, IoT devices, and enterprise software.
Once collected, this data goes through a cleaning and processing phase. That means removing duplicates, correcting errors, and formatting it for machine consumption. Clean data is essential because AI models are only as good as the information they’re trained on—garbage in, garbage out.
In the U.S., companies use powerful data lakes and warehouses to store and manage this data. AI then analyzes it to detect patterns, spot trends, and make predictions. This foundational step is critical—without accurate data collection and processing, the AI pipeline simply won’t work.
Model Training and Testing
After collecting and processing data, the next step is training the AI model. Think of this like teaching a new employee. The AI system is given labeled data (for supervised learning) and shown examples of how it should respond.
For instance, if the goal is to detect spam emails, the system is fed thousands of examples of both spam and non-spam emails. It learns the subtle differences, like specific keywords, formatting quirks, or sender behavior.
Once trained, the model is tested against a new set of data to see how accurately it can perform. This validation phase helps refine the model. Sometimes, models go through dozens of iterations before they’re considered reliable enough for deployment.
In American businesses, data scientists work alongside AI engineers to tune these models, balancing accuracy with speed and ensuring they align with business goals.
Real-Time Decision Making
One of the main advantages of AI is its ability to make real-time decisions. Once a model is trained, it can process incoming data and deliver instant decisions. Whether it involves adjusting product prices, recommending content, detecting fraud, or routing a delivery, AI can respond in milliseconds.
For example, Uber’s AI systems analyze ride requests, driver locations, traffic conditions, and surge pricing models all in real-time to connect you with a driver in seconds.
These decisions are driven by complex algorithms but are designed to seem seamless to the end user. The AI doesn’t just act fast—it acts smart, adapting continuously based on new data and feedback.
Integration with Business Systems
To truly be effective, AI must be integrated with a company’s existing tools and platforms. This includes CRM systems like Salesforce, eCommerce platforms like Shopify, or enterprise systems like SAP.
API integrations and cloud-based AI services make this easier than ever. Tools like Microsoft Azure, Google Cloud AI, and Amazon Web Services (AWS) allow businesses to plug in AI capabilities without having to build them from scratch.
In the USA, businesses of all sizes—from startups to Fortune 500 companies—are embedding AI into their digital infrastructure. The key is making sure AI doesn’t work in a silo but rather becomes an invisible force powering everything from customer interactions to backend logistics.
Real-World Examples of AI in U.S. Businesses
Amazon’s AI-Powered Personalization
Amazon is perhaps the poster child for AI in retail. Its recommendation engine uses AI to analyze what you buy, search, and review. Then, it makes incredibly accurate suggestions that often result in more sales. This level of personalization has helped Amazon achieve sky-high conversion rates and customer retention.
Beyond recommendations, Amazon also uses AI in its fulfillment centers. Robots and machine learning algorithms optimize inventory placement, packing, and shipping—helping reduce delivery times and operational costs.
Tesla’s Autonomous Driving System
Tesla’s self-driving technology is another brilliant example of AI in action. Its Autopilot feature uses neural networks trained on millions of miles of driving data. Cameras, sensors, and radar collect real-time information about the environment, which AI processes to navigate roads, avoid obstacles, and make driving decisions.
Though still under development, Tesla’s use of AI has already transformed automotive expectations. In the USA, Tesla’s system is considered a benchmark for the future of autonomous mobility.
Netflix’s Recommendation Engine
Netflix doesn’t just throw movies at you—it curates them using AI. Its recommendation engine analyzes what you’ve watched, how you rated shows, what you skipped, and what you rewatched. The algorithm then creates a unique homepage for every user.
This personalization has helped Netflix keep viewers engaged, reduce churn, and dominate the streaming market. AI is the silent but powerful brain behind your binge-watching habits.
JPMorgan’s AI for Fraud Detection
In the finance sector, JPMorgan Chase has deployed AI across various fronts. Its COiN platform reviews legal documents and extracts key data points much faster than humans. The company also uses AI algorithms to monitor transactions for fraudulent behavior in real time.
By identifying anomalies quickly, the system can block suspicious activity before it causes damage. JPMorgan’s use of AI improves accuracy, compliance, and customer trust—vital elements in the financial world.
Challenges and Limitations of AI in Business
Data Privacy and Ethical Concerns
With great power comes great responsibility—and AI is no exception. One of the biggest concerns in the U.S. business world is data privacy. AI systems rely on large datasets, many of which contain sensitive personal information. Improper handling can lead to violations of privacy laws like the California Consumer Privacy Act (CCPA) or even lawsuits.
Ethical concerns also arise when AI decisions affect people’s lives—like approving loans, hiring employees, or sentencing in court systems. Bias in AI models, often inherited from biased training data, can lead to unfair or discriminatory outcomes.
High Initial Investment
AI promises long-term savings, but the upfront costs can be steep. Developing custom models, hiring data scientists, and integrating AI into legacy systems requires significant investment. For many small to mid-sized businesses in the USA, this is a major hurdle.
That said, cloud-based AI services and pre-built models are making AI more accessible. Still, cost remains a key barrier to widespread adoption.
Lack of Skilled Workforce
Despite the growing interest in AI, there’s a shortage of skilled professionals. Data scientists, AI engineers, and machine learning specialists are in high demand but in short supply. U.S. companies are competing fiercely to attract and retain top AI talent.
This talent gap limits how quickly and effectively businesses can adopt and scale AI technologies.
Algorithm Bias and Fairness Issues
AI systems learn from historical data, and this data can contain human biases. In areas such as facial recognition, hiring platforms, and financial services, biased algorithms can produce skewed or discriminatory results.
While U.S. regulators are starting to tackle these issues, it is also essential for businesses to take responsibility. Implementing transparent algorithms, using diverse training data, and conducting regular audits are important steps toward ensuring fairness in AI.
Future of AI in American Business
Emerging Trends in AI
The future of AI in U.S. business looks both promising and transformative. One of the most exciting trends is the rise of generative AI—systems that can create content, code, music, and even design. Tools like ChatGPT, DALL·E, and GitHub Copilot are redefining how work gets done, enabling professionals to boost productivity and creativity like never before.
Another trend is the growing importance of AI ethics and explainability. As AI systems take on more decision-making power, businesses are under pressure to ensure these decisions are transparent, fair, and accountable. Explainable AI (XAI) is becoming a necessity, especially in regulated sectors like healthcare, finance, and law.
We’re also seeing the democratization of AI. No longer limited to tech giants, even small businesses are beginning to access AI tools thanks to low-code and no-code platforms. Services like Zapier and OpenAI’s API are bringing powerful capabilities to non-tech-savvy entrepreneurs, leveling the playing field in innovative ways.
Finally, AI at the edge—using AI in local devices rather than central servers—is picking up steam. Think smart security systems, wearable health monitors, or autonomous delivery bots. This reduces latency, improves privacy, and allows for quicker decision-making.
Policy and Regulatory Landscape
As AI continues to influence more areas of life and business, U.S. lawmakers are racing to catch up. Regulations like the California Privacy Rights Act (CPRA) and national efforts like the AI Bill of Rights reflect growing concern over how data is used, stored, and monetized.
Expect stricter compliance requirements around AI transparency, bias testing, and user consent shortly. Companies will need to build AI systems that are not only effective but also explainable and ethical.
At the federal level, there are proposals for national AI standards and frameworks, aimed at fostering innovation while ensuring accountability. The National Institute of Standards and Technology (NIST) has already released draft frameworks that businesses may soon be required to follow.
This regulatory shift will push businesses to rethink how they design, deploy, and govern AI technologies, creating opportunities for compliance-focused innovation.
The Role of AI in Sustainability
AI isn’t just about profit—it can also drive positive environmental and social change. Many U.S. companies are using AI to reduce carbon emissions, optimize energy use, and promote sustainability.
For example, AI helps in energy management by predicting consumption patterns and automating power grids. It’s being used to monitor deforestation, track wildlife, and even predict climate disasters before they strike.
In manufacturing, AI improves resource allocation, minimizing waste and energy consumption. In agriculture, AI analyzes soil health, weather data, and crop performance to help farmers make better decisions, reducing the environmental impact of food production.
As ESG (Environmental, Social, and Governance) goals become more important to investors and consumers, AI will play a pivotal role in helping businesses meet these benchmarks.
Conclusion
Artificial Intelligence (AI) has transformed from a theoretical concept into a powerful force driving innovation across nearly every industry in the United States. It enhances customer service, streamlines operations, revolutionizes healthcare and transforms transportation. AI is not just a trend; it is a business necessity.
The U.S. stands out due to its unique combination of resources: significant investment in research, a robust tech ecosystem, renowned academic institutions, and a culture that embraces change. This environment enables American businesses to lead the global AI revolution.
However, with this power comes great responsibility. As AI becomes more integrated into our daily lives and business processes, issues such as ethics, transparency, and fairness must be prioritized. How we address these challenges will not only affect the success of individual companies but also influence public trust in this transformative technology.
For tech startups, legacy manufacturers, and small business owners alike, the message is clear: integrating AI is no longer a question of “if,” but “when.” Those who act early and strategically will secure a significant competitive advantage in an increasingly AI-driven world.
FAQs
What is AI technology in business terms?
AI technology in business refers to the use of intelligent systems and algorithms that can mimic human cognition to automate tasks, analyze data, and make decisions. This includes everything from chatbots and virtual assistants to complex data analytics platforms used for forecasting and planning.
How do U.S. businesses implement AI?
U.S. businesses implement AI through various means—using cloud-based AI platforms, integrating AI APIs into their software, and deploying machine learning models trained on business-specific data. Most implementations begin with pilot projects in areas like customer service, marketing, or logistics.
Which industries benefit most from AI?
Industries such as healthcare, finance, retail, manufacturing, logistics, and tech see the most significant benefits. Each uses AI in unique ways—ranging from predictive diagnostics in healthcare to fraud detection in finance and personalized shopping in retail.
Are there downsides to using AI in business?
Yes, challenges include high implementation costs, data privacy issues, workforce displacement, and potential biases in decision-making. Additionally, a lack of transparency in some AI models can make it hard to understand or explain how decisions are made.
What’s the future outlook for AI in the USA?
The future of AI in the U.S. is incredibly promising. With advancements in generative AI, ethical frameworks, and democratization through low-code tools, we’ll see AI embedded in nearly every aspect of business. Expect more regulations, more innovation, and more mainstream adoption in the years to come.
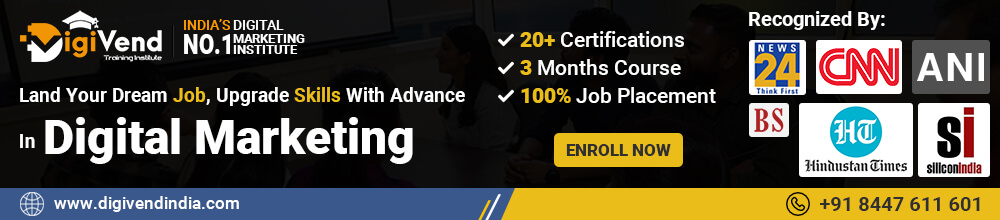