Comprehensive Guide to Predictive Maintenance: Transforming Asset Management

In today’s fast-paced industrial landscape, maximizing asset efficiency and minimizing downtime are paramount to staying competitive. Predictive maintenance (PdM) has emerged as a game-changing strategy that leverages advanced technologies to anticipate equipment failures before they occur, ensuring uninterrupted operations and cost savings. This comprehensive guide delves into the intricacies of predictive maintenance, its benefits, applications, and the role of maintenance software in transforming asset management.
Understanding Predictive Maintenance
Predictive maintenance is a proactive approach that utilizes data analysis tools and techniques to detect anomalies and predict equipment failures. Unlike reactive maintenance (fixing equipment after it breaks) and preventive maintenance (routine inspections and servicing), PdM focuses on forecasting issues and addressing them before they escalate. By analyzing data from various sources, such as sensors, historical records, and machine learning algorithms, PdM identifies patterns that signal potential malfunctions.
Key Components of Predictive Maintenance
- Data Collection: The foundation of PdM lies in collecting real-time data from equipment through sensors, Internet of Things (IoT) devices, and other monitoring tools. This data includes temperature, vibration, pressure, and other critical parameters.
- Data Analysis: Advanced analytics, including machine learning and artificial intelligence (AI), process the collected data to identify trends, anomalies, and correlations that indicate potential failures.
- Condition Monitoring: Continuous monitoring of equipment conditions allows for early detection of issues. This involves tracking key performance indicators (KPIs) and setting thresholds to trigger alerts.
- Prediction Models: Predictive algorithms create models that forecast when a component is likely to fail. These models consider historical data, operational conditions, and environmental factors to provide accurate predictions.
- Maintenance Scheduling: Based on the predictions, maintenance activities are scheduled at optimal times to prevent failures, reduce downtime, and extend the lifespan of assets.
Benefits of Predictive Maintenance
Implementing predictive maintenance offers numerous advantages for businesses across various industries:
- Reduced Downtime: By predicting failures before they happen, PdM minimizes unplanned downtime, ensuring continuous operations and productivity.
- Cost Savings: Preventing catastrophic failures and optimizing maintenance schedules reduces repair costs, labor expenses, and inventory carrying costs.
- Increased Equipment Lifespan: Regular monitoring and timely interventions extend the life of equipment, maximizing return on investment (ROI).
- Enhanced Safety: Early detection of potential hazards minimizes the risk of accidents, protecting workers and the environment.
- Improved Efficiency: PdM enhances operational efficiency by optimizing maintenance tasks and resource allocation, leading to smoother workflows.
Applications of Predictive Maintenance
Planned maintenance is applicable across a wide range of industries, each benefiting from tailored solutions:
- Manufacturing: PdM ensures continuous production by monitoring critical machinery, reducing production line stoppages, and improving product quality.
- Energy and Utilities: Power plants, wind farms, and other energy facilities use PdM to maintain equipment reliability, prevent outages, and optimize energy production.
- Transportation: Railways, airlines, and logistics companies leverage PdM to keep vehicles and infrastructure in top condition, reducing delays and maintenance costs.
- Healthcare: Medical equipment, such as MRI machines and ventilators, benefit from PdM by ensuring they are always operational, enhancing patient care.
- Oil and Gas: PdM helps monitor drilling rigs, pipelines, and refineries, preventing costly failures and ensuring safe operations.
Technologies Enabling Planned Maintenance
Several advanced technologies underpin the success of predictive maintenance:
- Internet of Things (IoT): IoT devices and sensors collect real-time data from equipment, providing a constant stream of information for analysis.
- Big Data Analytics: The vast amount of data generated by IoT devices is processed using big data analytics to identify patterns and trends.
- Machine Learning (ML): ML algorithms analyze historical data to create predictive models that forecast equipment failures with high accuracy.
- Artificial Intelligence (AI): AI enhances PdM by enabling intelligent decision-making, anomaly detection, and automated responses.
- Cloud Computing: Cloud platforms provide the necessary storage, processing power, and accessibility for managing large datasets and running complex analyses.
- Digital Twins: Digital twins are virtual replicas of physical assets that simulate real-time conditions, allowing for precise predictions and scenario testing.
Implementing Planned Maintenance: A Step-by-Step Guide
Implementing planned maintenance requires a structured approach to ensure successful adoption and integration:
- Assess Needs and Objectives: Identify critical assets and define the goals of the PdM initiative, such as reducing downtime or extending equipment lifespan.
- Data Collection Strategy: Determine the data sources and types of sensors needed to monitor equipment conditions effectively.
- Choose the Right Tools: Select predictive maintenance software and analytics platforms that align with your needs and infrastructure.
- Data Integration: Integrate data from various sources into a centralized system for comprehensive analysis.
- Develop Predictive Models: Use historical data and machine learning algorithms to create predictive models tailored to your equipment.
- Pilot Testing: Conduct pilot tests on a subset of equipment to validate the predictive models and refine the approach.
- Full-Scale Deployment: Roll out the planned maintenance program across all relevant assets, ensuring proper training and support for maintenance teams.
- Continuous Improvement: Regularly review and update predictive models based on new data and insights to enhance accuracy and effectiveness.
Challenges and Solutions in Predictive Maintenance
While planned maintenance offers significant benefits, it also presents certain challenges:
- Data Quality: Ensuring high-quality, accurate data is crucial for reliable predictions. Regular calibration and maintenance of sensors are essential.
- Integration Complexity: Integrating data from diverse sources and systems can be complex. Adopting standardized protocols and using compatible tools can ease this process.
- Cost: Initial implementation costs can be high. However, the long-term savings and ROI justify the investment.
- Skill Gaps: Implementing PdM requires specialized skills in data analysis and machine learning. Training and hiring experts can bridge this gap.
- Change Management: Transitioning to a predictive maintenance approach requires a cultural shift within the organization. Clear communication and demonstrating the benefits can facilitate this change.
Case Studies: Successful Predictive Maintenance Implementation
Case Study 1: General Electric (GE)
General Electric implemented planned maintenance across its aviation division, leveraging IoT and big data analytics to monitor engine health. By predicting potential failures and optimizing maintenance schedules, GE reduced unscheduled maintenance events by 25% and achieved significant cost savings.
Case Study 2: Siemens
Siemens applied predictive maintenance to its industrial machinery, using digital twins and AI to simulate real-time conditions. This approach resulted in a 20% reduction in maintenance costs and a 30% decrease in downtime, enhancing overall productivity.
Case Study 3: Delta Airlines
Delta Airlines adopted predictive maintenance for its fleet, utilizing sensors and machine learning to monitor aircraft components. This initiative led to a 98% reduction in unscheduled maintenance, improving flight reliability and customer satisfaction.
Conclusion
Predictive maintenance is transforming the way industries manage their assets, offering unprecedented opportunities for efficiency, cost savings, and reliability. As technology continues to evolve, the integration of predictive maintenance software will become increasingly sophisticated, enabling even more accurate predictions and automated maintenance actions.
By harnessing the power of predictive maintenance software, businesses can stay ahead of potential issues, ensuring seamless operations and maintaining a competitive edge in today’s dynamic market. Investing in PdM not only safeguards assets but also paves the way for a future where downtime is minimized, costs are controlled, and productivity is maximized.
Embrace the future of asset management with predictive maintenance and experience the transformative impact it can have on your operations.
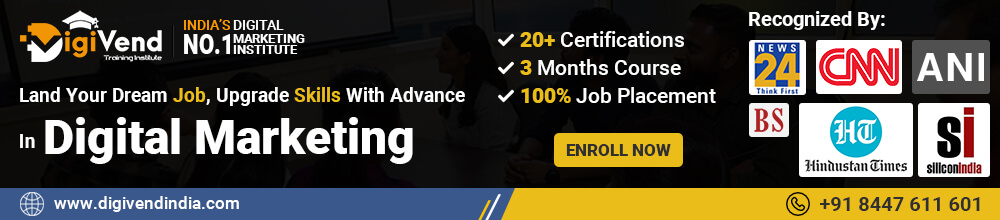