The Potential of AI in Drug Discovery In the realm
In the realm of pharmaceuticals, the integration of Artificial Intelligence (AI) is ushering in a new era of drug discovery. The traditional drug development process is time-consuming and resource-intensive, often taking years to bring a new drug to market. AI, with its capacity for rapid data analysis and pattern recognition, is transforming this landscape. This article delves into the immense potential of AI in drug discovery, exploring its applications, benefits, and the revolutionary impact it holds for the future of medicine.
The Imperative for Innovation in Drug Discovery
The need for innovative drugs to address complex diseases necessitates a paradigm shift in drug discovery. AI offers a powerful solution by expediting the identification of potential drug candidates, streamlining the research process, and enhancing the efficiency of clinical trials.
Accelerated Target Identification
AI algorithms analyze vast biological datasets to identify potential drug targets rapidly. By understanding the genetic and molecular basis of diseases, AI helps researchers pinpoint specific proteins or genes that can be targeted for therapeutic intervention.
Validation through Data Integration
AI integrates diverse datasets, including genomics, proteomics, and clinical data, to validate potential drug targets. This comprehensive approach enhances the understanding of disease mechanisms, increasing the likelihood of successful target validation.
Rational Drug Design
AI aids in rational drug design by predicting the interactions between drugs and target molecules. Machine learning models analyze molecular structures and predict the binding affinity of potential drug candidates, facilitating the selection of compounds with higher chances of success.
De Novo Drug Discovery
AI enables de novo drug discovery, where novel molecules are designed from scratch. Generative models use learned patterns to propose new chemical structures, potentially uncovering drug candidates that traditional methods might overlook.
Repurposing Existing Drugs
Identification of Drug Repurposing Opportunities
AI leverages large-scale data analysis to identify existing drugs with the potential to treat different diseases. By repurposing drugs, researchers can bypass extensive safety testing, accelerating the availability of treatment options for various conditions.
Predicting Drug Combinations
AI models predict synergistic drug combinations for enhanced therapeutic effects. This approach is particularly valuable in cancer treatment, where combination therapies can target multiple pathways, overcoming resistance and improving treatment outcomes.
Patient Stratification
AI analyzes patient data to stratify populations based on genetic, molecular, and clinical characteristics. This enables more precise recruitment for clinical trials, ensuring that participants are more likely to respond positively to the treatment.
Predictive Analytics for Trial Outcomes
AI models use historical clinical trial data to predict outcomes, including potential side effects and patient responses. This predictive analytics approach helps optimize trial design, reducing costs and increasing the chances of successful trials.
Data Quality and Bias
The reliance on vast datasets introduces challenges related to data quality and bias. Ensuring that datasets used for AI in drug discovery are representative and free from biases is essential for reliable results.
Interpretability of AI Models
Interpreting the decisions made by AI models remains a challenge. Understanding how AI arrives at specific conclusions is crucial for gaining trust in its recommendations, especially in highly regulated industries like pharmaceuticals.
Cross-Industry Collaborations
The future of AI in drug discovery involves increased collaboration between pharmaceutical companies, technology firms, and research institutions. These partnerships can pool resources, share data, and collectively advance the field.
Advances in Explainable AI
Efforts to enhance the interpretability of AI models are ongoing. Developments in explainable AI aim to make the decision-making processes of these models more transparent, addressing concerns related to trust and accountability.
Conclusion
AI’s potential in drug discovery represents a transformative force in the pharmaceutical industry. From identifying novel drug targets to streamlining clinical trials, AI accelerates the pace of drug development, offering new hope for faster, more efficient, and innovative solutions to address global health challenges.
FAQs
How does AI contribute to target identification in drug discovery? AI contributes to target identification by analyzing biological datasets to rapidly identify potential drug targets. By understanding the genetic and molecular basis of diseases, AI aids in pinpointing specific proteins or genes for therapeutic intervention.
What is rational drug design, and how does AI assist in it? Rational drug design involves predicting interactions between drugs and target molecules. AI assists in rational drug design by analyzing molecular structures and predicting the binding affinity of potential drug candidates, facilitating the selection of compounds with higher chances of success.
How does AI enable de novo drug discovery? AI enables de novo drug discovery by designing novel molecules from scratch. Generative models use learned patterns to propose new chemical structures, potentially uncovering drug candidates that traditional methods might overlook.
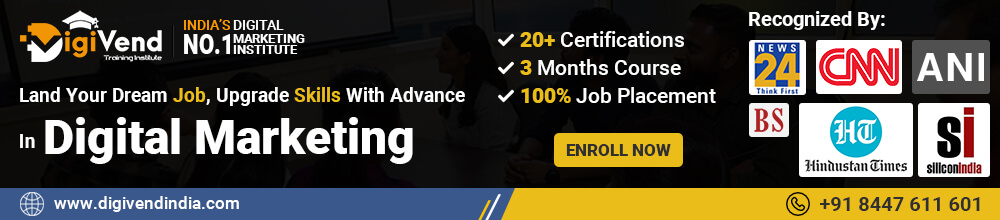