Understanding Data Analysis in Online Surveys: Making Sense of Responses
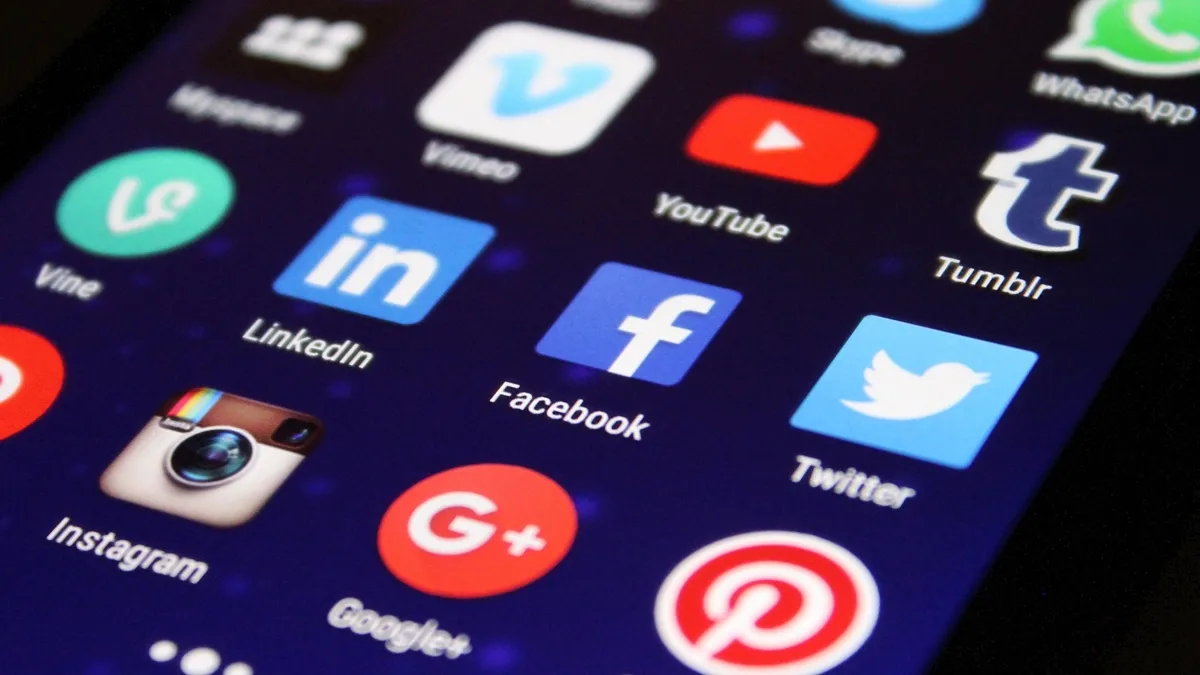
Introduction
In the realm of online surveys, collecting data is just the first step. Making sense of the collected data through effective analysis is crucial for extracting actionable insights and informing decision-making processes. This article aims to delve into the intricacies of data analysis in online surveys, from fundamental concepts to advanced techniques, to help researchers and analysts unlock the full potential of survey responses.
Fundamentals of Data Analysis
Data analysis involves examining, cleaning, transforming, and interpreting data to uncover patterns, trends, and relationships. Key concepts include measures of central tendency (mean, median, mode), variability (standard deviation, variance), and distribution.
Cleaning and Preparing Data
Before analysis can begin, it’s essential to clean and prepare the data. This involves identifying and removing duplicates, outliers, and missing values, as well as formatting the data in a consistent manner for ease of analysis.
Descriptive Statistics
Descriptive statistics provide a summary of the main features of a dataset. Measures such as mean, median, mode, standard deviation, and variance offer insights into the central tendency and variability of the data.
Data Visualization Techniques
Data visualization techniques, such as bar charts, histograms, scatter plots, and pie charts, provide graphical representations of survey data. Visualizations aid in understanding patterns and relationships within the data and are instrumental in conveying findings to stakeholders.
Interpreting Survey Data
Interpreting survey data involves identifying trends, patterns, and outliers that may inform decision-making. It requires a deep understanding of the survey objectives and context to draw meaningful insights from the data.
Quantitative vs. Qualitative Analysis
Quantitative analysis focuses on numerical data and employs statistical methods to identify relationships and correlations. Qualitative analysis, on the other hand, involves interpreting textual or narrative data to uncover themes, opinions, and sentiments.
Statistical Analysis Methods
Statistical analysis methods, such as correlation analysis and regression analysis, help researchers understand the relationships between variables and make predictions based on survey data.
Segmentation and Filtering
Segmentation involves dividing survey data into subsets based on demographic or other criteria to analyze responses more granularly. Filtering allows researchers to focus on specific subsets of data for targeted analysis. Using a reliable survey maker, you can segment the users based on their responses which helps in better decision-making.
Advanced Analysis Techniques
Advanced analysis techniques, including factor analysis and cluster analysis, enable researchers to uncover complex relationships and groupings within the data.
Ensuring Data Accuracy and Reliability
Ensuring data accuracy and reliability is paramount in data analysis. This involves validating survey responses, verifying data integrity, and conducting sensitivity analyses to assess the robustness of findings.
Interpreting Open-Ended Responses
Interpreting open-ended responses requires coding and categorizing textual data to extract meaningful insights. Text analysis tools and techniques can aid in systematically analyzing large volumes of text data.
Combining Survey Data with Other Sources
Integrating survey data with other sources, such as customer relationship management (CRM) or enterprise resource planning (ERP) systems, enhances analysis by providing additional context and insights.
Challenges and Pitfalls in Data Analysis
Challenges in data analysis include data quality issues, sample bias, and the complexity of interpreting qualitative data. Overcoming these challenges requires robust methodologies, careful validation, and interdisciplinary collaboration.
Conclusion
In conclusion, data analysis plays a pivotal role in the online survey process, serving as the bridge between raw data and actionable insights. Through a thorough understanding of fundamental concepts and the adept application of various analytical techniques, researchers can extract valuable information from survey responses. However, the journey from data collection to meaningful interpretation is not without its challenges.
One significant challenge is ensuring the quality and reliability of the data collected. Researchers must employ strategies to minimize bias, such as random sampling and carefully crafted survey questions, to obtain accurate representations of the target population. Additionally, dealing with missing or incomplete data requires thoughtful handling techniques to avoid compromising the integrity of the analysis.
Moreover, the vast amount of data generated by online surveys can be overwhelming without proper tools and methodologies in place for analysis. Analysts may need to utilize data visualization techniques to simplify complex datasets and facilitate comprehension. Additionally, the integration of advanced analytical tools, such as machine learning algorithms, can uncover hidden patterns and trends within the data that may not be immediately apparent through traditional analysis methods.
Despite these challenges, the insights gleaned from data analysis are invaluable for informing decision-making processes and driving organizational success. By harnessing the power of data, researchers can gain a deeper understanding of their target audience, identify emerging trends, and make informed strategic decisions that align with their objectives. Ultimately, data analysis serves as a cornerstone of the online survey process, empowering researchers to unlock the full potential of survey data and drive meaningful change.
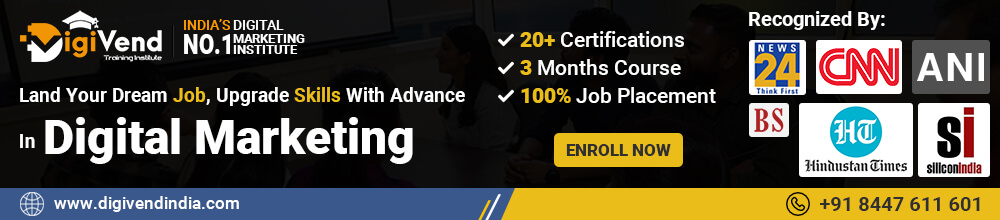