AI in Finance: From Algorithmic Trading to Fraud Detection
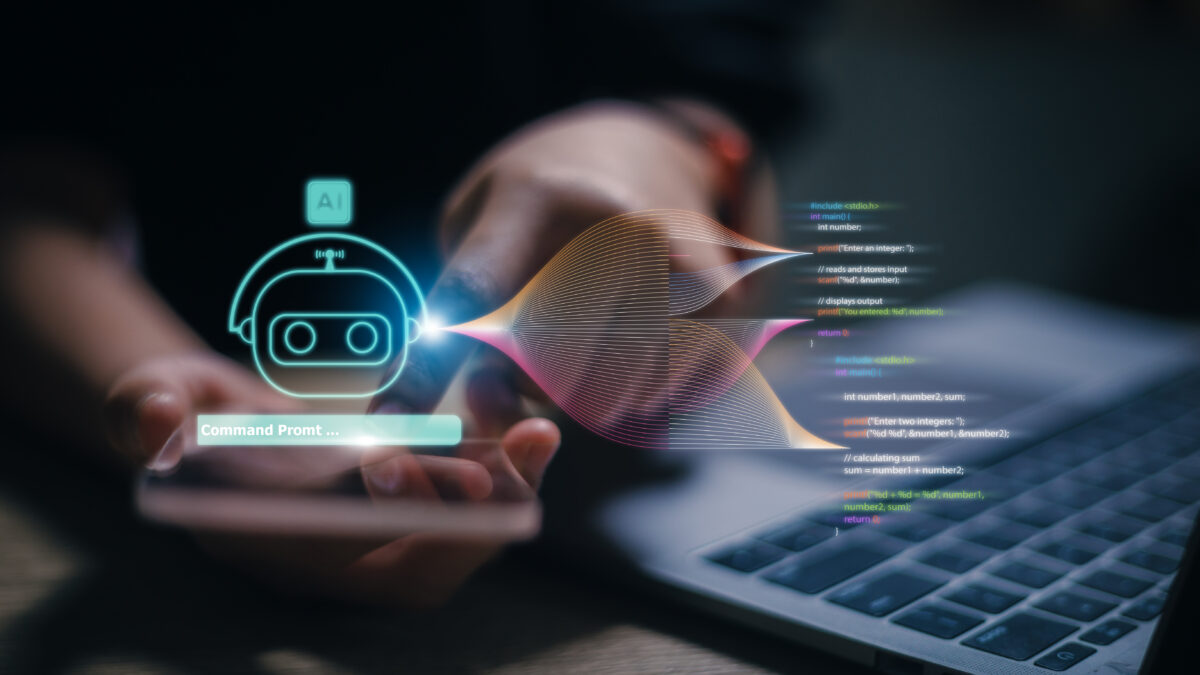
Introduction
The financial industry is undergoing a paradigm shift with the infusion of Artificial Intelligence (AI) into its diverse domains. From algorithmic trading strategies fueled by machine learning models to advanced fraud detection systems, AI is reshaping the financial landscape. In this article, we delve into the technical aspects and profound implications of AI in finance while also exploring the role of certifications, such as the Global Tech Council’s AI certification, in shaping the expertise of professionals.
I. Algorithmic Trading: Unleashing the Power of Machine Learning
A. The Rise of Algorithmic Trading
Algorithmic trading, or algo trading, stands as a testament to the transformative impact of AI in financial markets. AI algorithms, often supported by certifications like the AI engineer certification, analyze vast datasets at speeds unattainable by human traders. This evolution highlights the importance of continuous learning through certifications to stay abreast of the latest AI developments.
B. Machine Learning in Algorithmic Trading
1. Predictive Modeling
Machine learning algorithms, a cornerstone of algorithmic trading, are instrumental in predictive modeling. Professionals armed with AI certifications, such as the AI expert certification, are well-versed in leveraging historical data to forecast future price movements, employing techniques like regression analysis and time series forecasting.
2. Neural Networks in Trading
Certified AI developers play a pivotal role in implementing neural networks within algorithmic trading. Deep learning models, including recurrent neural networks (RNNs) and long short-term memory networks (LSTMs), enhance the predictive capabilities of algorithms, contributing to the success of AI-driven trading strategies.
3. Reinforcement Learning for Trading Strategies
Professionals holding AI certifications are well-equipped to deploy reinforcement learning in developing adaptive trading strategies. The continuous optimization of algorithms based on rewards and penalties ensures that AI-driven trading systems remain dynamic and responsive to changing market conditions.
C. Challenges and Risks in Algorithmic Trading
1. Overfitting and Model Complexity
Certified AI professionals are keenly aware of challenges like overfitting in algorithmic trading models. Striking a balance between model complexity and generalization, a skill honed through certifications like the AI developer certification, is crucial for the development of robust and resilient trading algorithms.
2. Market Dynamics and Unforeseen Events
Certified professionals, with their expertise validated through AI certifications, navigate the challenges posed by sudden market shifts and unforeseen events. The Flash Crash of 2010 serves as a stark reminder of the need for algorithms to be resilient, and continuous learning through certifications ensures that professionals are adept at managing such risks.
II. Credit Scoring and Loan Approval: AI’s Impact on Risk Assessment
A. Traditional Credit Scoring vs. AI-powered Models
1. The Evolution of Credit Scoring
The integration of AI in credit scoring is transforming risk assessment practices. AI certifications, like those offered by the Global Tech Council, equip professionals to augment traditional credit scoring with advanced machine learning techniques, incorporating alternative data sources beyond the scope of conventional credit reports.
2. Alternative Data and Feature Engineering
Professionals with AI certifications understand the significance of alternative data and adeptly employ feature engineering. AI algorithms, scrutinizing data beyond credit reports—analyzing social media activity and online behavior—enhance predictive power. This holistic approach is a hallmark of professionals holding AI certifications.
B. Explainability and Ethical Concerns in Credit Scoring AI
1. The Black Box Dilemma
The lack of interpretability in AI models for credit scoring poses challenges, and professionals with certifications in AI, such as the Global Tech Council’s AI certification, are at the forefront of addressing the black box dilemma. Clear insights into decision-making processes are essential to meet regulatory requirements and ethical standards.
2. Bias and Fairness in Credit Scoring AI
Certified AI experts actively address biases inherent in training data, mitigating the risk of discriminatory outcomes in credit decisions. Regulatory bodies emphasize fairness, and professionals with AI certifications play a pivotal role in ensuring that AI algorithms contribute to fair lending practices.
III. Fraud Detection: AI’s Role in Safeguarding Financial Systems
A. The Escalating Threat of Financial Fraud
As financial transactions move into the digital realm, the threat of fraud necessitates advanced solutions. AI, with its real-time data analysis capabilities, emerges as a powerful tool in the fight against fraudulent activities, and certified professionals play a crucial role in implementing and maintaining these systems.
B. Machine Learning for Fraud Detection
1. Anomaly Detection
AI professionals, certified in areas like chatbot development, leverage machine learning models for anomaly detection in fraud prevention systems. Techniques such as clustering and isolation forests, coupled with the expertise gained through certifications, enhance the accuracy of identifying and mitigating potential fraudulent transactions.
2. Behavioral Biometrics
Certified experts in AI, particularly those with chatbot certifications, contribute to the arsenal of fraud detection methods by incorporating behavioral biometrics. Analysis of user behavior patterns, including keystroke dynamics and mouse movements, adds an extra layer of security, protecting financial systems from unauthorized access and fraudulent activities.
Generative AI: Surge in Talent, Tools, and Lucrative Careers
C. Real-time Monitoring and Adaptive Systems
Certified AI professionals implement real-time monitoring in fraud detection systems, continuously learning from new data to stay ahead of emerging threats. This adaptive approach, a hallmark of certified experts, ensures the effectiveness of fraud detection systems in an ever-changing landscape.
IV. Regulatory Compliance and Explainability: Navigating the Legal Landscape
A. The Regulatory Framework for AI in Finance
1. Striking a Balance
The integration of AI in finance necessitates a delicate balance between innovation and consumer protection, a challenge that certified professionals, grounded in the regulatory landscape through certifications like those offered by the Global Tech Council, are well-prepared to navigate.
2. Explainability Requirements
Certified AI professionals address the regulatory emphasis on explainability, providing transparency into the decision-making processes of complex algorithms. Meeting these requirements, a key focus of certifications, ensures accountability and compliance with regulatory standards.
B. Legal and Ethical Implications of AI in Finance
1. Data Privacy and Security
AI professionals, certified and well-versed in data privacy regulations, ensure robust measures to protect sensitive information. Certifications, such as those from the Global Tech Council, equip professionals to navigate legal landscapes, including compliance with the General Data Protection Regulation (GDPR).
2. Fair Lending and Discrimination Concerns
Certified AI experts play a pivotal role in addressing fair lending and discrimination concerns. The ethical considerations ingrained in certifications guide professionals to develop and deploy AI algorithms that contribute to fair lending practices, thereby meeting regulatory standards.
V. The Future Landscape of AI in Finance
A. Integration of Quantum Computing
Certified AI professionals anticipate the integration of quantum computing into the financial sector. Quantum algorithms, a focus of ongoing certifications, have the potential to revolutionize algorithmic trading, optimizing portfolio management, and enhancing risk assessment strategies.
B. Explainable AI Advancements
Certified AI professionals, with a commitment to ongoing learning through certifications, champion advancements in explainable AI (XAI). Clearer insights into decision-making processes enhance trust among consumers and financial institutions, addressing the challenges associated with the lack of transparency in complex algorithms.
C. Collaboration Between Financial Institutions and Fintech
The collaborative efforts between traditional financial institutions and fintech companies, often led by certified professionals, accelerate the adoption of AI in finance. Fintech startups, armed with innovative solutions, collaborate with established institutions, fostering a symbiotic relationship that propels the evolution of AI in finance.
Conclusion
As AI continues to shape the financial landscape, its integration into algorithmic trading, credit scoring, and fraud detection is propelled by certified professionals. The Global Tech Council’s AI certifications, along with ongoing learning initiatives, contribute to the expertise required to navigate challenges and strike a balance between innovation and ethical considerations. In this era of artificial intelligence, certifications play a pivotal role in defining the future of finance and ensuring the responsible and effective use of AI technologies.
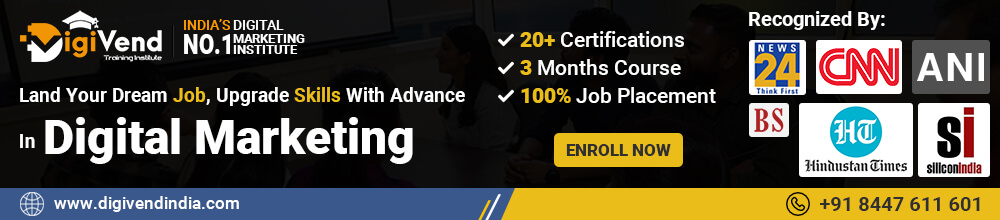